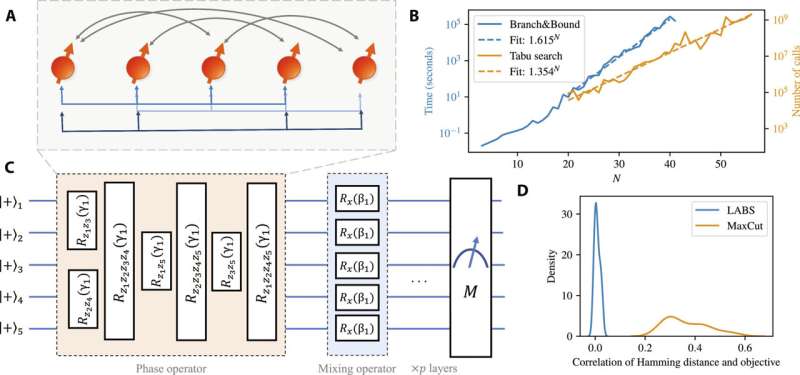
Classical and quantum algorithms applied to the LABS problem. Credit: Advances in science (2024). DOI: 10.1126/sciadv.adm6761
In a new letter in Advances in scienceresearchers at JPMorgan Chase, the US Department of Energy’s (DOE) Argonne National Laboratory, and Quantinuum have demonstrated clear evidence of a quantum algorithmic speedup for the quantum approximate optimization algorithm (QAOA).
This algorithm has been widely studied and implemented in many quantum computers. It has potential applications in fields such as logistics, telecommunications, financial modeling and materials science.
“This work is an important step toward achieving the quantum edge, laying the groundwork for future impact on manufacturing,” said Marco Pistoia, head of Global Applied Technology Research at JPMorgan Chase.
The team examined whether a quantum algorithm with low implementation costs could provide a quantum speedup over the more popular classical methods. QAOA was applied to the problem of Binary Sequences with Low Autocorrelation, which is important in understanding the behavior of physical systems, signal processing, and cryptography. The study showed that if the algorithm was asked to tackle increasingly large problems, the time it would take to solve them would increase at a slower rate than that of a classical solver.
To explore the performance of the quantum algorithm in an ideal noise-free environment, JPMorgan Chase and Argonne jointly developed a simulator to evaluate the algorithm’s performance at scale.
“Large-scale quantum circuit simulations efficiently utilized the DOE petascale Polaris supercomputer housed at the ALCF. These results demonstrate how high-performance computing can complement and advance the field of quantum information science,” said Yuri Alexeev, a computational scientist at Argonne. Jeffrey Larson, a computational mathematician in Argonne’s Division of Mathematics and Computer Science, also contributed to this research.
To take the first step toward realizing the speedup in the algorithm in practice, the researchers demonstrated a small-scale implementation on the quantum computers with trapped ions of the Quantinuum Model H1 and H2. Using algorithm-specific error detection, the team reduced the impact of errors on algorithmic performance by up to 65%.
“Our long-standing partnership with JPMorgan Chase led to this important and meaningful three-pronged research experiment that also brought Argonne. The results could not have been achieved without the unprecedented and world-leading quality of our H-series quantum computer, which provides a flexible device for running debugging and error detection experiments at top gate fidelity that is years ahead of other quantum computers,” said Ilyas Khan, Quantinuum’s founder and chief product officer.
More information:
Ruslan Shaydulin et al, Proof of scaling advantage for approximate quantum optimization algorithm in a hard classical problem, Advances in science (2024). DOI: 10.1126/sciadv.adm6761
Provided by Argonne National Laboratory
citation: Research team demonstrates theoretical quantum speedup with approximate quantum optimization algorithm (2024, May 29) Retrieved May 30, 2024 from https://phys.org/news/2024-05-team-theoretical-quantum-speedup-approximate .html
This document is subject to copyright. Except for any fair agreement for study or private research purposes, no part may be reproduced without written permission. The content is provided for informational purposes only.
#research #team #demonstrates #theoretical #quantum #speed #approximate #quantum #optimization #algorithm
Image Source : phys.org